Problem:
Users have a range of different opinions and express them in different ways. Where machine learning content detection is used to flag and hide offensive comments left online, users want their comments treated as fairly as anyone else’s.
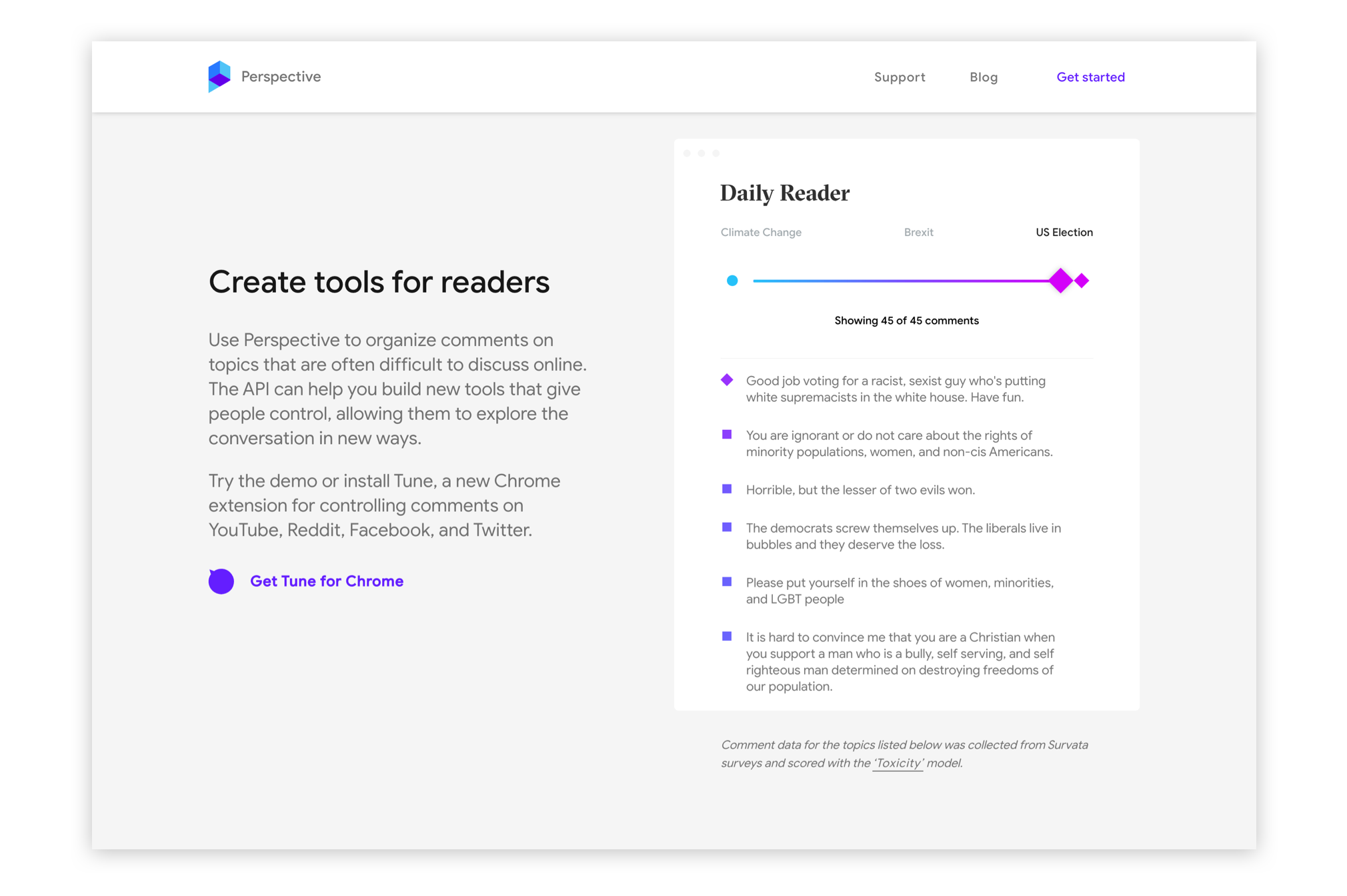
Anti-pattern response:
The content filter flags up some reasonable comments as toxic while letting through other genuinely toxic comments, due to its inability to understand the meaning behind the comment or through other biases designed into the system. At best this is randomly unfair, but at worst this bias aligns with political orientations, privileging one group and discriminating against another.
Discussion:
While the filtering of offensive content and policing of toxic behaviour online is badly needed, few organizations are willing to invest in the workforce required to manually moderate these spaces. As such, tools that automate the process could be revolutionary in improving the everyday experience for people online. Unfortunately, as is common in AI and ML systems, it is easy to accidentally or inconsiderately replicate existing biases that leave some people feeling like they are treated unfairly.
For example, from the tech demo of Perspective, an experimental ML content flagging system project from Jigsaw and Google, the following comments about the 2016 US election results were flagged as toxic:
“It was terrible. Both sides suck, but Trump REALLY is scary.”
“You are ignorant or do not care about the rights of minority populations, women, and non-cis Americans.”
“Please put yourself in the shoes of women, minorities, and LGBT people.”
On the other hand, the following were passed as non-toxic:
“Your [sic] a socialist snowflake!”
“Great. We need our country back!”
“Make America Great Again!”
While this latter group might not use profane language, they are well established sayings understood to express triumphalism over political opponents, intimidate minorities, and be generally divisive.
The question is then, by what measure is a comment “toxic”? Can further training help tweak this and create a fairer system? Or is it inevitable that the system appears to be unfair to one party or another?